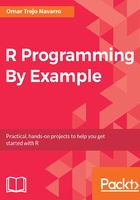
Setting up the data
As it's usual with data analysis, the first step is to understand the data we will be working with. In this case, the data is the same as in Chapter 2, Understanding Votes with Descriptive Statistics, and we have already understood some of its main characteristics. Mainly, we've understood that age, education, and race have considerable effects over the propensity to vote in favor of the UK leaving or remaining in the EU.
The focus of this chapter will be on using linear models to predict the Proportion and Vote variables, which contain the percentage of votes in favor of leaving the EU and whether the ward had more votes for "Leave" or "Remain", respectively. Both variables have similar information, the difference being that one is a numerical continuous variable with values between 0 and 1 (Proportion) and the other is a categorical variable with two categories (Vote with Leave and Remain categories).
We'll keep observations that contain complete cases in the data object, and observations that have missing values for the Proportion and Vote variables in the data_incomplete object (we'll make predictions over these in the latter part of this chapter). The functions prepare_data(), adjust_data(), and get_numerical_variables() come from Chapter 2, Understanding Votes with Descriptive Statistics, so you may want to take a look if you're not clear about what they do. Basically, they load the data with the adjusted version that we created by compressing the data spread among various variables regarding age, education, and race:
data <- adjust_data(prepare_data("./data_brexit_referendum.csv")) data_incomplete <- data[!complete.cases(data), ] data <- data[ complete.cases(data), ] numerical_variables <- get_numerical_variable_names(data)