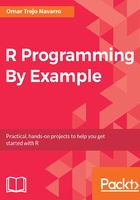
Programming by visualizing the big picture
Now, we will work with a top-down approach, meaning that we'll start with abstract code first and gradually move into the implementation details. Generally I find this approach to be more efficient when you have a clear idea of what you want to do. In our case, we'll start by working with the main.R file.
The first thing to note is that we will use the proc.time() function twice, once at the beginning and once at the end, and we will use the difference among these two values to measure how much time it took for the whole code to execute.
The second thing to note is that the empty_directories() function makes sure each of the specified directories exist, and deletes any files contained in them. We use it to clean up our directories at the beginning of each execution, to make sure we have the latest files, and only the files created in the last run. The actual code is shown below, and it simply iterates through each of the directories passed, removes any files inside recursively with the unlink() function, and makes sure the directory exists with the dir.create() function. It avoids showing any warnings due to the directory already existing, which is not a problem in our case, by using the showWarnings = FALSE parameter.
empty_directories <- function(directories) {
for (directory in directories) {
unlink(directory, recursive = TRUE)
dir.create(directory, showWarnings = FALSE)
}
}
From Chapter 1, Introduction to R, we use of the print_section() and empty_directories() functions to print headers and delete directory contents (to re-create the results every time we run the function with empty directories), respectively, and we'll use the mechanism shown with proc.time() to measure execution time.
Now that the previous two points are out of the way, we proceed to show the full contents of the main.R file.
start_time <- proc.time() source("./functions.R") empty_directories(c( "./results/original/", "./results/adjusted/", "./results/original/scatter_plots/" )) data <- prepare_data("./data_brexit_referendum.csv", complete_cases = TRUE) data_adjusted <- adjust_data(data) numerical_variables <- get_numerical_variable_names(data) numerical_variables_adj <- get_numerical_variable_names(data_adjusted) print("Working on summaries...") full_summary(data, save_to = "./results/original/summary_text.txt") numerical_summary( data, numerical_variables = numerical_variables, save_to = "./results/original/summary_numerical.csv" ) print("Working on histograms...") plot_percentage( data, variable = "RegionName", save_to = "./results/original/vote_percentage_by_region.png" ) print("Working on matrix scatter plots...") matrix_scatter_plots( data_adjusted, numerical_variables = numerical_variables_adj, save_to = "./results/adjusted/matrix_scatter_plots.png" ) print("Working on scatter plots...") plot_scatter_plot( data, var_x = "RegionName", var_y = "Proportion", var_color = "White", regression = TRUE, save_to = "./results/original/regionname_vs_proportion_vs_white.png" ) all_scatter_plots( data, numerical_variables = numerical_variables, save_to = "./results/original/scatter_plots/" ) print("Working on correlations...") correlations_plot( data, numerical_variables = numerical_variables, save_to = "./results/original/correlations.png" ) print("Working on principal components...") principal_components( data_adjusted, numerical_variables = numerical_variables_adj, save_to = "./results/adjusted/principal_components" ) end_time <- proc.time() time_taken <- end_time - start_time print(paste("Time taken:", taken[1])) print("Done.")
As you can see, with just this file, you get the big picture of the analysis, and are able to reproduce your analysis by running a single file, save the results to disk (note the save_to arguments), and measure the amount of time it takes to perform the full analysis. From our general objectives list, objectives one through four are fulfilled by this code. Fulfilling objectives five and six will be accomplished by working on the functions.R file, which contains lots of small functions. Having this main.R file gives us a map of what needs to be programmed, and even though right now it would not work because the functions it uses do not yet exist, by the time we finish programming them, this file will not require any changes and will produce the desired results.
Due to space restrictions, we won't look at the implementation of all the functions in the main.R file, just the representative ones: prepare_data(), plot_scatter_plot(), and all_scatter_plots(). The other functions use similar techniques to encapsulate the corresponding code. You can always go to this book's code repository (https://github.com/PacktPublishing/R-Programming-By-Example) to see the rest of the implementation details. After reading this book, you should be able to figure out exactly what's going on in every file in that repository.
We start with prepare_data(). This function is abstract and uses four different concrete functions to do its job, read.csv(), clean_data(), transform_data(), and, if required, complete.cases(). The first function, namely read.csv(), receives the path to a CSV file to read data from and loads into a data frame object named data in this case. The fourth function you have seen before in Chapter 1, Introduction to R, so we won't explain it here. Functions two and three are created by us, and we'll explain them. Note that main.R doesn't know about how data is prepared, it only asks for data to be prepared, and delegates the job to the abstract function prepare_data().
prepare_data <- function(path, complete_cases = TRUE) { data <- read.csv(path) data <- clean_data(data) data <- transform_data(data) if (complete_cases) { data <- data[complete.cases(data), ] } return(data) }
The clean_data() function simply encapsulates the re-coding of -1 for NA for now. If our cleaning procedure suddenly got more complex (for example, new data sources requiring more cleaning or realizing we missed something and we need to add it to the cleaning procedure), we would add those changes to this function and we would not have to modify anything else in the rest of our code. These are some of the advantages of encapsulating code into functions that communicate intention and isolate what needs to be done into small steps:
clean_data <- function(data) { data[data$Leave == -1, "Leave"] <- NA return(data) }
To transform our data by adding the extra Proportion and Vote variables, and re-label the region names, we use the following function:
transform_data <- function(data) { data$Proportion <- data$Leave / data$NVotes data$Vote <- ifelse(data$Proportion > 0.5, "Leave", "Remain") data$RegionName <- as.character(data$RegionName) data[data$RegionName == "London", "RegionName"] <- "L" data[data$RegionName == "North West", "RegionName"] <- "NW" data[data$RegionName == "North East", "RegionName"] <- "NE" data[data$RegionName == "South West", "RegionName"] <- "SW" data[data$RegionName == "South East", "RegionName"] <- "SE" data[data$RegionName == "East Midlands", "RegionName"] <- "EM" data[data$RegionName == "West Midlands", "RegionName"] <- "WM" data[data$RegionName == "East of England", "RegionName"] <- "EE" data[data$RegionName == "Yorkshire and The Humber", "RegionName"] <- "Y" return(data) }
All of these lines of code you have seen before. All we are doing is encapsulating them into functions that communicate intention and allow us to find where certain procedures are taking place so that we can find them and change them easily if we need to do so later on.
Now we look into plot_scatter_plot(). This function is between being an abstract and a concrete function. We will use it directly in our main.R file, but we will also use it within other functions in the functions.R file. We know that most of the time we'll use Proportion as the color variable, so we add that as a default value, but we allow for the user to remove the color completely by checking if the argument was sent as FALSE, and since we will use this same function to create graphs that resemble all the scatter plots we have created up to this point, we will make the regression line optional.
Note that in the case of the former graphs, the x axis is a continuous variable, but in the case of the latter graph, it's a categorical (factor) variable. This kind of flexibility is very powerful and is available to us due to ggplot2's capability to adapt to these changes. Formally, this is called polymorphism, and it's something we'll explain in Chapter 8, Object-Oriented System to Track Cryptocurrencies.
Finally, instead of assuming the user will always want to save the resulting graph to disk, we make the save_to argument optional by providing an empty string for it. When appropriate, we check to see if this string is empty with not_empty(), and if it's not empty, we set up the PNG saving mechanism.
plot_scatter_plot <- function(data, var_x, var_y, var_color = "Proportion", regression = FALSE, save_to = "") { if (var_color) { plot <- ggplot(data, aes_string(x = var_x, y = var_y, color = var_color)) } else { plot <- ggplot(data, aes_string(x = var_x, y = var_y)) } plot <- plot + scale_color_viridis() plot <- plot + geom_point() if (regression) { plot <- plot + stat_smooth(method = "lm", col = "grey", se = FALSE) } if (not_empty(save_to)) png(save_to) print(plot) if (not_empty(save_to)) dev.off() }
Now we look into all_scatter_plots(). This function is an abstract function that hides from the user's knowledge the name of the function that will create graphs iteratively, conveniently named create_graphs_iteratively(), and the graphing function, the plot_scatter_plot() function we saw before. In case we want to improve the iterative mechanism or the graphing function, we can do so without requiring changes from people that use our code, because that knowledge is encapsulated here.
Encapsulate what changes frequently or is expected to change.
The create_graphs_iteratively() function is the same we have seen before, except for the progress bar code. The progress package provides the progress_bar$new() function that creates a progress bar in the terminal while an iterative process is being executed so that we see what percentage of the process has been completed and know how much time is remaining (see Appendix, Required Packages for more information).
Note the change in the save_to argument from the functions plot_scatter_plot() and all_scatter_plots(). In the former, it's a filename; in the latter, a directory name. The difference is small, but important. The incautious reader might not notice it and it may be a cause for confusion. The plot_scatter_plot() function produces a single plot, and thus receives a file name. However, the all_scatter_plots() will produce, by making use of plot_scatter_plot(), a lot of graphs, so it must know where all of them need to be saved, create the final image names dynamically, and send them one-by-one to plot_scatter_plot(). Finally, since we want the regression to be included in these graphs, we just send the regression = TRUE parameter:
all_scatter_plots <- function(data, numerical_variables, save_to = "") { create_graphs_iteratively(data, numerical_variables, plot_scatter_plot, save_to) } create_graphs_iteratively <- function(data, numerical_variables, plot_function, save_to = "") {
numerical_variables[["Proportion"]] <- FALSE variables <- names(numerical_variables[numerical_variables == TRUE]) n_variables <- (length(variables) - 1) progress_bar <- progress_bar$new( format = "Progress [:bar] :percent ETA: :eta", total = n_variables ) for (i in 1:n_variables) { progress_bar$tick() for (j in (i + 1):length(variables)) { image_name <- paste( save_to, variables[i], "_", variables[j], ".png", sep = "" ) plot_function( data, var_x = variables[i], var_y = variables[j], save_to = image_name, regression = TRUE ) } } }
The other functions that we have not looked at in detail follow similar techniques as the ones we showed, and the full implementation is available at this book's code repository (https://github.com/PacktPublishing/R-Programming-By-Example).